“The magic of driving insights from data” is the title of a recent presentation by a well-known speaker in the world of analytics.
Well… the thing is I never see my peers or myself as magicians (though my son would be thrilled to find out his dad has magical powers!).

The problem with “magic” is that it cultivates unrealistic expectations. Joshua Althauser from explains this really well in a recent TNW article:
A company’s eventual success is based on a variety of factors, and data analysis should be treated as simply one of those vital factors, rather than a magic solution.
We all love labels and superheroes, like rockstars and magicians and ninjas and so on, but none of those labels do any justice to data scientists. If anything, they increase the disconnect between data and business.
[clickToTweet tweet=”Truth being told, entrepreneurs don’t believe in magic – at all #saas #metrics” quote=”Truth being told, entrepreneurs don’t believe in magic – at all. #saas #metrics”]
You don’t need to be a sorcerer or a mighty wizard to drive insights from data. You just need the right mindset and toolset.
After all, it’s called data science, not data magic. Here is how data driven companies do it:
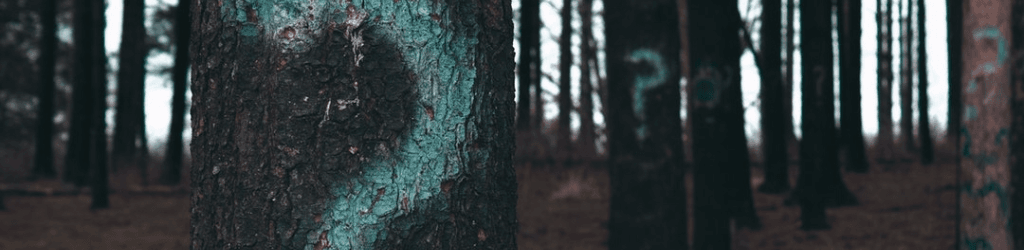
Start with a question (hypothesis)
Everyone has an opinion, so it’s time to use that to your advantage. I think it’s best when hypotheses come in the shape of questions.
So, what is the question that, once answered, will lead you towards a decision?
Still too theoretical? Ok, let’s try an example: Is the quality of traffic from paid ads worse than the one from organic sources?

Map out scenarios
In other words, what scenarios can come out of data and how would you go about them?
Let’s play with our example:
Scenario 1: The traffic from paid ads is of low quality.We should check the quality performance of each individual campaign. We will focus on the low performing campaigns and work towards getting them at the same level as the high performing ones.
Scenario 2: If the traffic from paid ads is of high quality, we should focus on content that non-paid traffic is interacting with. (change SEO strategy, partnerships, content).
Action paths for each scenario are clear. We can move to the next stage.
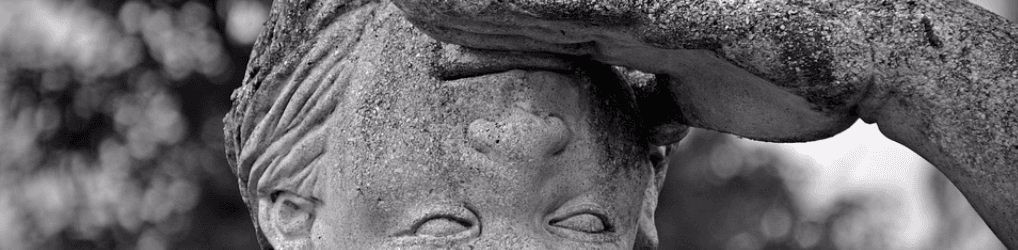
Invalidate scenarios
We need to figure out what data we need to invalidate each scenario.
First we need to define what “quality” is for traffic coming from paid ads. One way to do it is to define it based on the conversion rates from signup to onboarding, to the 1st week retention and eventually to payment.
We need to identify the quality goals for each paid advertising campaign and see if it is above or below the overall conversion rates for non-paid traffic.
Now that the strategy and tactics are set, let’s get to work and see what tools can help us answer our question.
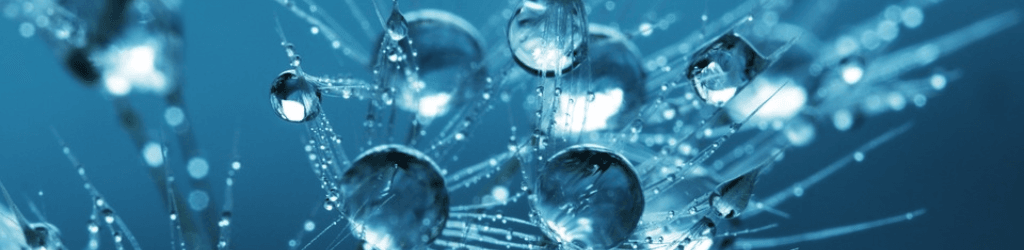
Aggregate, clean and filter your data
We need to link each paid ad click to a signup and then follow that signup across time and see how it performs.
Most users have multiple touch points to our website before they create an account. We need to know all of those touch points and link them to a signup. You can use this library for this purpose.
We need to make sure that, once a user signs up, we will be able to link the initial click to any interaction of that user regardless of how many devices he/she uses or how much time it takes to make them.
For that you will need to map the attribution data to your existing user-based analytics service which will link campaign attribution to user behavior.
For this you can use services like Tractionboard or InnerAdQ.
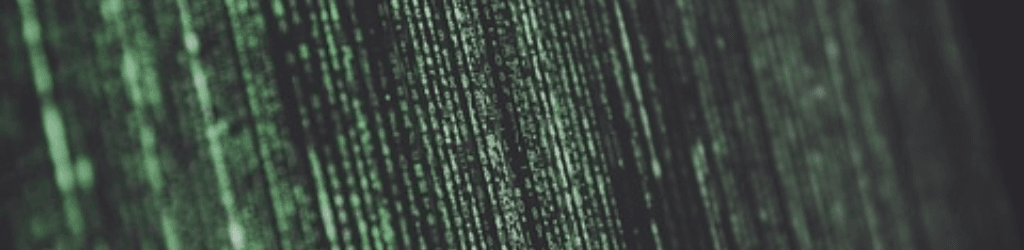
Apply data algorithms
It’s now that science comes in. Might look like magic to some but it’s just maths. Beautiful math.
In our case we will need to measure the time it takes for most users to get from signup to all the quality goals we are after.
That will define the timeframe needed to analyze the campaigns. If we have this wrong, we might be looking at partial data that is very likely to be biased.
If you measure the impact of a campaign for the 1st week retention before a week lapses since the start of the campaign, you’ll get 0 conversions.
Secondly we’ll need an algorithm that will help you identify statistically significant results. We can use the Chi-square test for this purpose. It’s easy to apply in an Excel sheet and there are tools that come with it integrated.

Visualize results & act
Is this a one time analysis? Excel will do just perfectly. Pull the data in it and add for each campaign clicks, leads, and how many of them reached the quality goals.
Order them by using a pivot table that considers both number of leads (quantity) and how many of them reached the goals you are after (quality). Apply CHI-square to identify the statistically significant results.
Need it on a regular basis? As mentioned earlier, services like InnerAdQ or Tractionboard are out of the box solutions for this problem.
Now that we have our results, we just go back to the scenarios we initially mapped for our hypothesis and act on them.
It’s not magic. It’s just science that anyone of you can do by following the above steps.
Your turn now! Does data science look like magic to you?
Looking for deep insights into how your customers use your product?
InnerTrends can help. You won’t have to be a data scientist to discover the best growth opportunities for your business, our software will take care of that for you.
Schedule a Demo with us and witness with your own eyes just how powerful InnerTrends can be.